“It’s humbling every time we train on years of effort”
Conjuring new science from the collective wisdom of the past
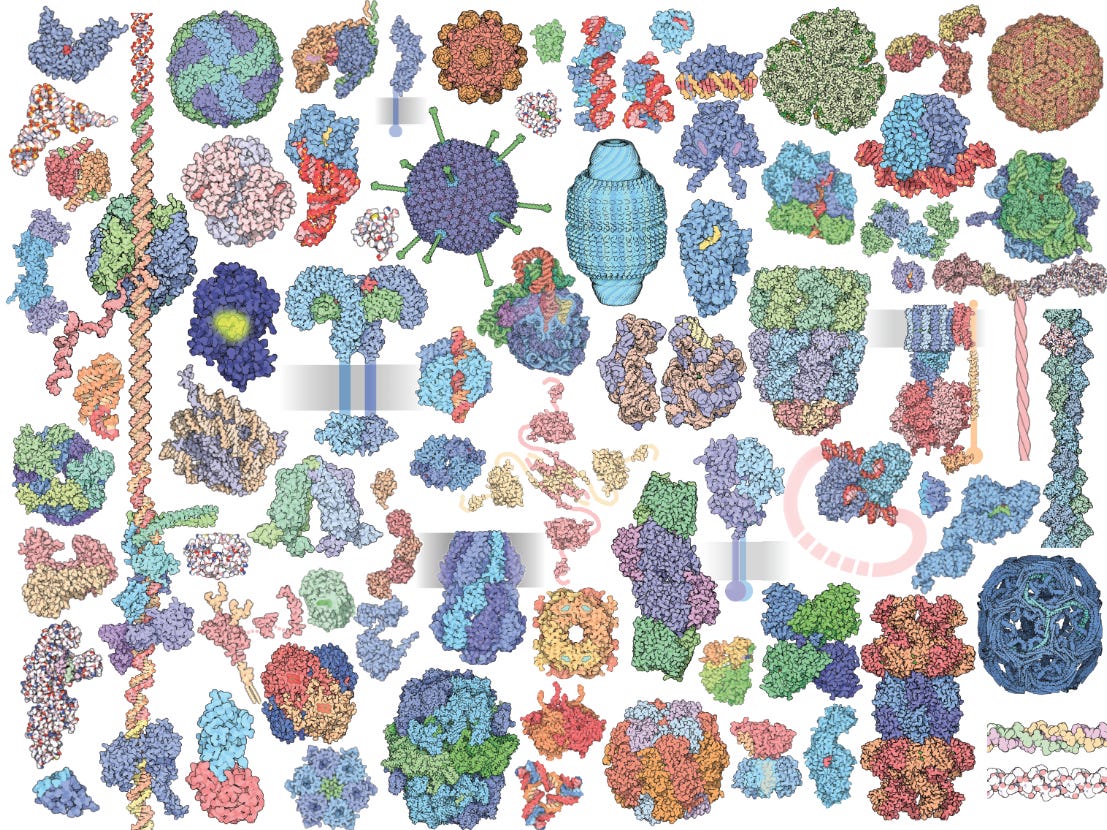
“Molecular biology is more magic and superstition than science.” This was how the PI who taught me to clone genes in *E. coli—*an organic chemist turned chemical biologist—started his lesson on how to transform cells.
Bunsen burner on while you’re plating your cells to protect from contamination? Magical thinking. Thirty seconds in the 42° water bath? Why not ten? Forty five? Zero? Thirty minute recovery before plating? Why not sixty? Five? Zero? Whatever worked for someone was handed down like an incantation in a spell book from one student to the next.
This early lesson helped me to critically evaluate protocols, build intuition for what matters and what doesn’t when working with DNA and microbes, and to cut the right corners and save time in my remaining years in the lab. But it also helped me to see those protocols as something much more profound—the product of painstaking, frustrating work, passed from student to student, lab to lab, handed down from one generation to another, connecting me to dense the web of others who seek to understand the living world.
Where my professor saw “unscientific” trial and error steeped in superstition, I felt the magic of collective knowledge transformed into the hard-won power to shape the living world. Science, after all, is magic that works.
I’m remembering this feeling more and more these days, a couple decades later, as the focus of conversation in science increasingly shifts to AI. In the wake of AI’s sweep of this year’s Nobels, journalist Ewen Callaway quoted AlphaFold winner John Jumper thanking the experimental community that made it possible to train their protein folding AI model. Of the incredible work that went into building the protein data bank of protein structures and sequences that trained their AI, he says:
“It’s humbling every time we train on years of effort. Each data point is years of effort from someone training to be a PhD student or for someone who has already gotten their PhD.”
Each structure that trained the model represents years of someone’s life. But/and it’s so much more than that—someone had to notice the crystals forming in samples of blood on glass slides. Someone had to figure out what those crystals were made out of. Someone had to figure out that they could diffract x-rays through these crystals if they suspended them just the right way (and someone had to figure out x-rays and instruments to produce them before that) and use those patterns to see the relationship between the atoms in the crystalline structure. Someone had to figure out the extremely precise and sometimes out-of-this-world conditions needed to purposely crystallize each different protein. Someone had to figure out how to curate and manage and store a database of all that knowledge and keep it free for researchers to use and to learn and create with.
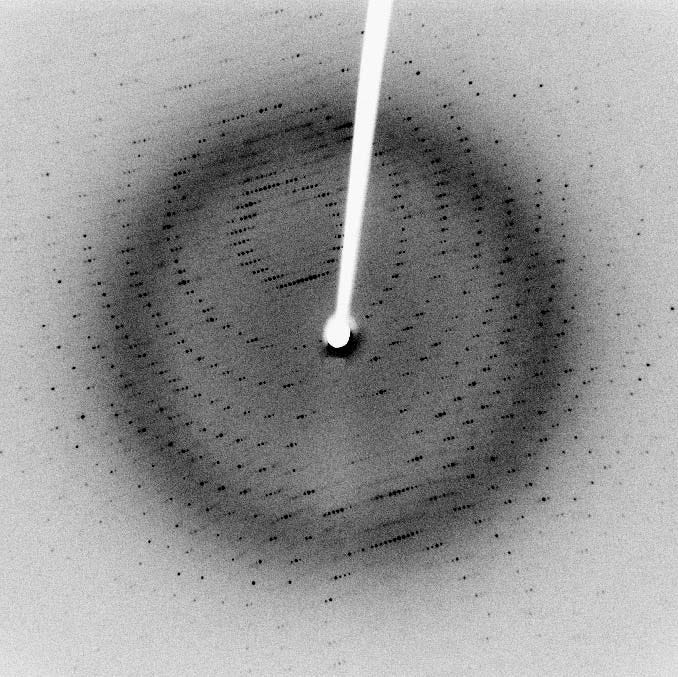
Divining the structure of a protein takes incredible dedication and years of training built on the collective insights of those who came before. Every protocol, instrument, dataset, hypothesis, everything that came before in every cited paper and all of those papers’ citations represent uncountable hours of work by people hoping to understand and build something interesting. AlphaFold is a monument to this collective effort, each new structure conjured in silicon an echo of all that came before.
Like other tools we train with in the lab, there is increasing evidence that these new tools themselves require hard-won domain knowledge, intuition, and critical judgement to be used to good effect. A recent paper from an MIT researcher looks at the impact of generative AI on the work of over 1000 materials scientists, finding that these tools significantly improved their productivity—but only if they were already among the most productive researchers in the cohort.
These experts who used AI most effectively had good judgement, able to “observe certain features of the materials design problem not captured by the algorithm” and evaluate the outputs of the model before spending time on experimental validation. Their practice of the craft gave them intuition about what matters and what doesn’t, and helps them choose from multiple paths and approaches to save time and find the most fruitful avenues.
The impact of these new tools is impossible to fully imagine today, but there’s something quite beautiful and important in the humility to recognize the collective intelligence they represent and the multiple intelligences they require and catalyze, just like the collective magic of science itself.
Totally agree with these algorithms being closer “collective” or inferred intelligence. I was thinking about that when a recent Nature paper claimed that AI-generated poetry could be better rated on metrics of beauty and rhythm than poets like Emily Dickinson and Chaucer. The model was trained on their work to start with! And then was essentially just using more accessible language.